2019_03_29_CIE_albareda
Complex networks and mathematics applied to the real world in the VI Albareda Lecture of the Chair TimacAgro-University of Navarre
The physicist Stefano Boccaletti, researcher of the Istituto dei Sistemi Complessi del Consiglio Nazionale della Richerche (CNRI) in Florence, was in charge of the lecture.
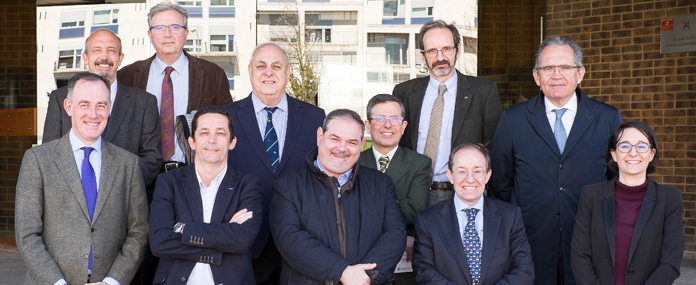
goal The VI Albareda Lecture, promoted by the Chair TimacAgro-University of Navarra with the aim of bringing relevant scientists in their respective fields to the university campus , was entitled degree scroll "Structure and function of complex networks: from applied mathematics to the real world". Taught by Dr. Stefano Boccaletti, researcher in the 90's in the incipient department of Physics and Applied Mathematics of campus Navarra, who, in the following years, has published more than 500 articles. He is currently researcher senior at the Istituto dei Sistemi Complessi del Consiglio Nazionale della Richerche (CNRI), Florence.
Dr. Bocaletti began the lecture by going back to graph theory and its applications, which has its birth in 1735, with Leonhard Euler's solution to the problem of the Seven Bridges of Königsberg, now Kaliningrad, in which by showing that one could not traverse all the regions of the city by crossing each of the bridges only once and returning to the starting point, he produced the first theorem in the field of graph theory and laid the instructions of modern complex network theory.
Two centuries later, in 1959, mathematicians Paul Erdös and Alfréd Rényi introduced a model for generating random graphs, which paved the way for proving the existence of several properties of random graphs in a rigorous way. This led to the central topic of Boccaletti's lesson: that although random graph models constitute a rigorous and elegant theory, real-world graphs are far from being considered random.
Just 6 steps can unite the entire U.S. population with one anotherIn the second part of the lecture, the guest physicist focused on the three most important properties that can be found in real and therefore complex networks: thesmall-world property, thescale-free property and themodularity property. Boccaletti described that, first of all, the graphs encountered when one wants to describe large groups of individuals establishing interpersonal interactions exhibit small-world properties. "In the late 1960s S. Milgram experiments examined the average length of shortest paths in social networks of people in the U.S. In such graphs, most individuals, called nodes, do not know each other (which in network jargon is said to be non-neighbors to each other), but the neighbors of any given node are likely to be neighbors to each other and, consequently, most nodes can be reached from any other node by a small issue steps." Specifically, in an average of only six steps.
Secondly, the availability of large amounts of data and the increase in computational capabilities, allowed the statistical study of complex networks: "A local measure of each node is its Degree, which consists of the issue of connections of that node. The Degrees distribution of the network tells us the probability of finding a node on the network with a given Degree ", explained the expert.
Finally, Dr. Boccaletti highlighted the property of modularity that exists in most real-world networks. This law consists in the fact that we can divide the network into different groups based on how the nodes are connected internally in each group and in relation to nodes in other groups. This concept is very relevant in the study of biological networks, such as networks of neurons in the brain.
Network theory to determine the genes causing a diseaseIn the third and last part of the lesson, Dr. Boccaletti explained the most important differences between the most common types of complex networks: physical, functional and parenclitic (from the Greek, deviation), focusing especially on the latter, which constitute one of his most important contributions.
The notion of structure of network, and the use of its analysis in terms of modules of tightly coupled groups of nodes, led to the introduction of his method, which converts groups of related information in many dimensions into networks where the nodes represent data featuresand the links are weighted according to the deviation (hence the name) between the values of two features and their corresponding baseline relationships obtained from a population of subjects.
This representation allows the identification of nodes causing systematic atypical conditions that, by other means, would be practically impossible to find. This subject of networks, as Dr. Boccaletti showed, has already been used to determine the genes that cause certain diseases, or those that determine the lesser or greater sensitivity of plants to abiotic stress (e.g., due to climate change).